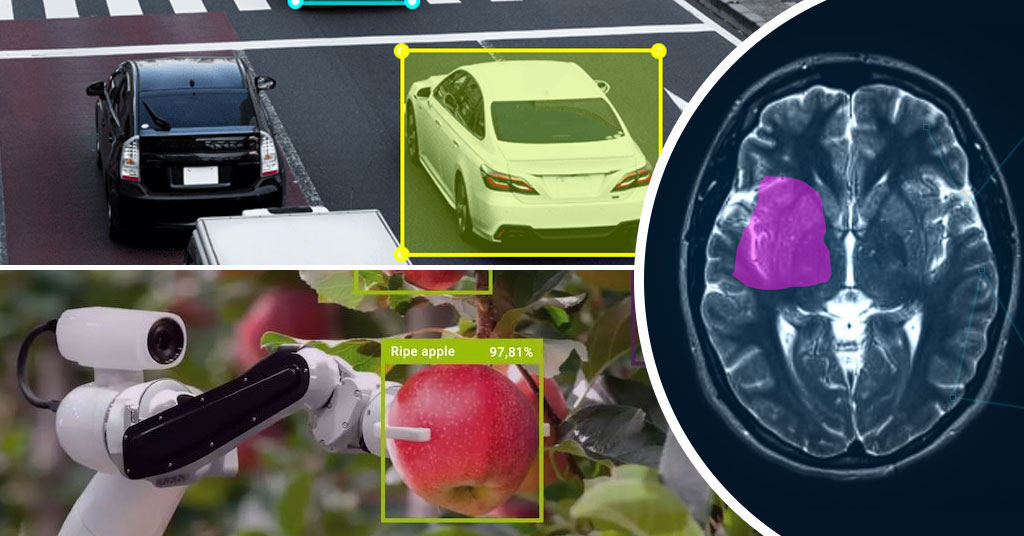
From healthcare to robotics, AI has made its way into every industry, automating workflows, personalizing user experiences, and enhancing operational efficiency. According to the National Center for Biotechnology Information (NCBI), using AI in healthcare can improve patient care and cut operational costs. It’s estimated that AI could save the US healthcare system about USD 150 billion by 2026. [Reference]. This surge in AI, particularly in vision-based applications, underscores the significance of image annotation. Accurately labeled image datasets are critical to developing and training robust AI/ML models that can revolutionize industries like healthcare, robotics, and autonomous vehicles. Let’s explore image annotation applications across these industries and the key considerations for ensuring the quality, security, and accuracy of annotated datasets.
Table of Contents
Image Annotation Applications in Healthcare, Robotics, and Autonomous Vehicle Domains
Image Annotation for Healthcare
AI/ML models, trained on high-quality annotated datasets, are revolutionizing healthcare through diverse applications ranging from early disease diagnosis to workflow automation. Key use cases include:
1. Disease Diagnosis and Treatment
- Medical Imaging & Prognosis
Anatomical structures in medical images such as X-rays, MRIs, and CT scans can be labeled to identify anomalies or abnormalities. These annotated images train AI models to detect and diagnose diseases (tumors, lesions, neurological disorders, etc.) more accurately at an early stage for effective treatment.
- Robotic Surgery
Annotated images are crucial for the development and refinement of robotic surgery models. By labeling key structures, such as organs, blood vessels, and abnormalities in medical images, robots can be programmed to assist surgeons or perform precise surgical procedures when needed.
- Drug Discovery and Development
In drug discovery, image annotation is essential to prepare high-quality labeled datasets necessary for analyzing cellular responses to various compounds. Molecular structures and biological images can be classified and labeled to train machine-learning models for identifying potential drug candidates, analyzing their interactions, and predicting their efficacy.
2. Workflow Optimization
- Document and Claim Management
Images related to patient records, treatment, and insurance data can be labeled to train computer vision models. These models can automate tasks like data extraction from forms and records, along with efficient data processing and management.
- Fraud Detection and Prevention
Annotated images help in detecting fraudulent activities by highlighting inconsistencies and unusual patterns in medical claims and records. This proactive approach protects healthcare resources and ensures legitimate claims are processed quickly.
3. Patient Monitoring
Visual data from medical devices and cameras can be annotated to train or develop advanced patient monitoring systems. These systems assist in tracking patient movements and monitoring their conditions in real-time to alert medical staff for immediate attention.
Image Annotation for Robotics
Image annotation is crucial for developing intelligent robotic systems that automate tasks across various industries. By labeling visual data, engineers can train robots to automate repetitive tasks, leading to increased efficiency and allowing human workers to focus on higher-level tasks. Robotics supports automation in diverse sectors such as biotechnology, agriculture, and manufacturing. Key applications of image annotation in robotics include:
1. Industrial Automation
- Quality control and inspection
Image annotation empowers AI systems to perform meticulous quality control that surpasses human capabilities. Trained on high-quality datasets, machine learning models can spot microscopic flaws on circuit boards, verify complex assemblies, check product labels, and assist in many other quality control processes.
- Defect detection in manufacturing
High-resolution images of products can be labeled using techniques like bounding boxes, polygons, or pixel-level labels to mark defects like cracks, scratches, and misalignments. Utilizing this annotated data, automated visual inspection systems can be developed and trained to efficiently detect manufacturing defects early, reducing waste and improving overall production efficiency.
2. Surveillance and Security
Skeletal and key point annotations help in creating training datasets for surveillance cameras to accurately monitor and identify potential human and animal threats. These systems can trigger alarms or lights when specific shapes are detected, providing real-time alerts. In case of a break-in, annotated data can assist law enforcement in identifying intruders through facial or body structure analysis. Advanced security cameras, equipped with motion tracking, can follow potential threats to avoid blind spots, ensuring comprehensive coverage
3. Agriculture
- Crop and Livestock Management
Annotated images train AI systems to identify different plant species and monitor crop health and livestock conditions. Leveraging the outcomes of the AI systems trained with context-driven image annotation, farmers can optimize farming practices and maintain the health of crops and animals with minimal human intervention.
- Geosensing
Annotated images from satellite and drone footage assist in geosensing applications, enabling precise mapping and analysis of agricultural lands. This information helps farmers make informed decisions about planting, irrigation, and harvesting.
- Unwanted crop detection
Image annotation is crucial in the development of AI systems used for identifying unwanted crops or weeds in fields. By annotating a large dataset of field images across different crop stages, lighting conditions, etc, AI systems can be trained to target specific areas for intervention, reducing herbicide use and promoting sustainable farming practices.
Image Annotation for Automotive
Image annotation plays a critical role in the development of autonomous driving systems for self-driving vehicles.
Key image annotation applications in automotive include:
1. Object Detection and Recognition
Advanced driver assistance systems and autonomous vehicles rely on annotated data for accurate object detection and recognition in diverse driving scenarios. By labeling vehicles, pedestrians, cyclists, traffic signs, and road markings, computer vision models can be trained for accurate object identification to avoid collisions and support path planning.
2. Environmental Understanding
Annotating road conditions, weather effects, time of day, and terrain features in images and video data allows autonomous vehicles to comprehend their surroundings, adjust to changing conditions, and anticipate potential risks.
3. In-Car Monitoring
In-car monitoring systems use annotated images to analyze driver behavior, such as attention levels, fatigue, and distraction, to mitigate potential risks and encourage safe driving practices.
Types of Image Annotation
Depending upon the use case, the annotation type required to create high-quality annotated datasets for AI/ML model training can vary. Some of the most popular types of image annotation are:
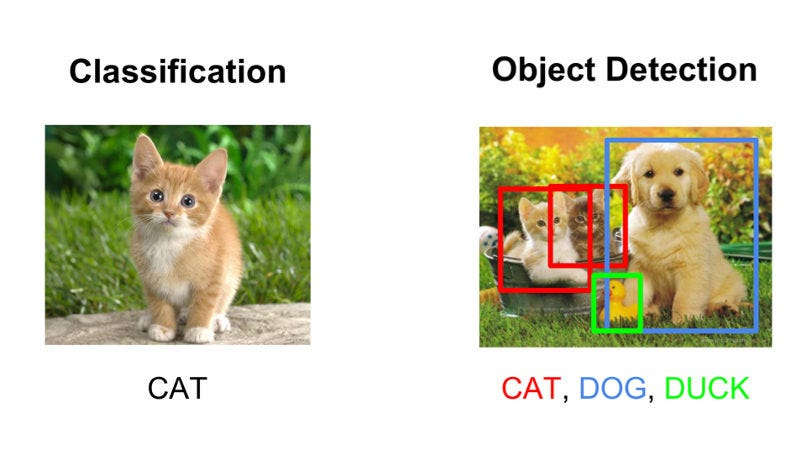
Image Classification
Involves categorizing an entire image into predefined classes or categories based on its content for object identification and pattern recognition. It can be helpful for automated organization of visual data, such as insurance companies classifying medical images such as healthy, diseased, or type of ailment for claims processing.
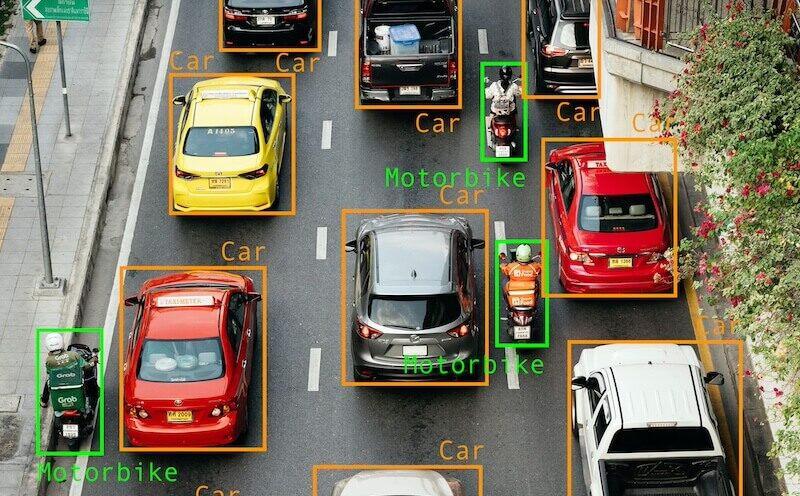
Bounding Boxes
Involves drawing rectangles (boxes) around objects of interest, such as cars on a road or products on a store shelf. It is essential for object detection and localization tasks, providing spatial coordinates that define the object’s position and size in the image.
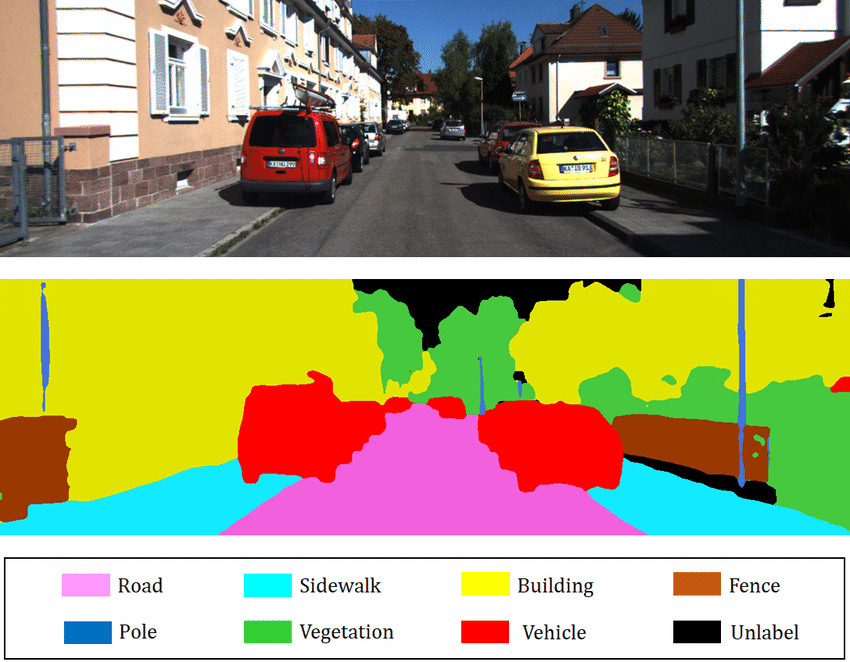
Semantic Segmentation
Involves assigning a semantic label to each pixel in an image, effectively dividing the image into meaningful parts. This technique is vital for applications like medical image analysis, where delineating organs or tissues accurately is critical for diagnosis and treatment planning.
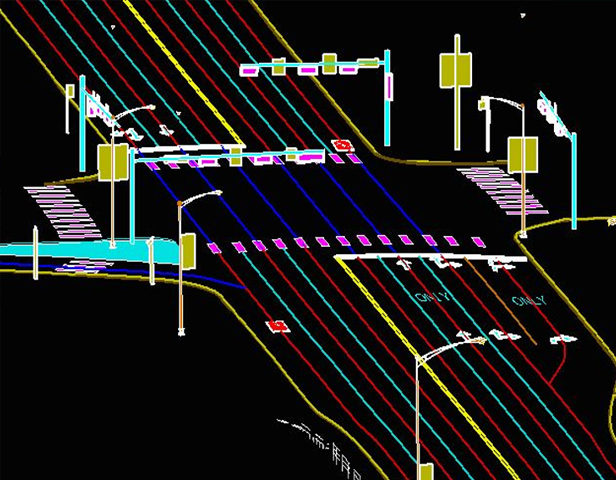
Polygon and Polyline Segmentation
Involves outlining irregular shapes or regions within an image using a connected series of straight lines (polylines) or closed shapes with straight sides (polygons). It is critical for urban planning and mapping, allowing for detailed analysis of satellite imagery.
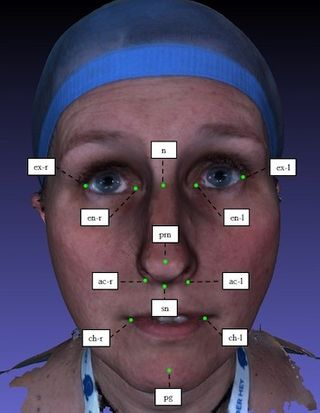
Landmarking
Involves identifying specific points of interest within an image, such as key anatomical features or distinctive landmarks. These points serve as reference markers for tasks like facial recognition, where identifying key facial landmarks (like eyes, nose, and mouth corners) enables precise alignment and analysis.
Challenges Involved in Image Annotation and their Possible Solutions
Image annotation, while crucial for AI model training, comes with its own set of challenges. Understanding and addressing these challenges head-on is essential for creating superior annotated datasets while upholding data security and process efficiency.
1. Data privacy and security concerns
Challenge: Images often contain sensitive information, such as faces, license plates, or personal belongings, raising concerns about privacy and security during annotation. Ensuring the confidentiality and integrity of sensitive data is paramount to complying with industry regulations such as HIPAA and GDPR.
Possible Solutions:
- Ensure annotators are aware of industry regulations and comply with them for data security and privacy.
- Utilize anonymization techniques (such as blurring or pixelation algorithms) to protect personally identifiable information (PII) in images.
- Employ data encryption techniques (e.g., AES for data at rest, TLS for data in transit).
- Implement access-based controls for data protection.
- Use secure annotation platforms with built-in security features.
2. Annotation quality and consistency
Challenge: Annotation can be subjective, with different annotators interpreting the same image differently. This subjectivity can lead to inconsistencies in annotations. Also, in case of a lack of subject matter expertise or clear image annotation guidelines, annotators can struggle to maintain consistency and accuracy.
Possible Solutions:
- Define detailed guidelines for image annotation based on use cases and project requirements and train annotators to follow them.
- Utilize annotation tools with built-in validation checks to ensure accuracy and consistency.
- Implement quality control measures such as double-checking annotations and using reference annotations to maintain high-quality standards.
3. Scalability and efficiency
Challenge: To build an intelligent system that can predict outcomes accurately and handle diverse scenarios, it is crucial to train AI models on large training datasets. Scaling annotation efforts to handle large datasets efficiently can be challenging for businesses, especially when tight deadlines or budget constraints are involved.
Possible Solutions:
- Automate tedious and repetitive tasks such as data organization, cleansing, and pre-processing utilizing automation and semi-automation tools for increased efficiency.
- Implement workflow optimizations such as task prioritization and parallel processing for large-scale image annotation.
- Employ a larger pool of annotators when needed to increase efficiency.
- Use cloud-based annotation platforms for scalability and flexibility in managing annotation tasks.
- Outsource image annotation services to a reliable third-party provider who has the required resources and expertise to accommodate your growing requirements.
Rely on the Integrated Approach for High-End Image Annotation – Human Expertise with Artificial Intelligence
Despite the advancements in machine learning algorithms and image annotation tools, their effectiveness is still limited by predefined rules and patterns. Human expertise is still irreplaceable and critical in image annotation, especially for the data validation phase. While automated annotation tools can enhance efficiency, subject matter experts can apply context, intuition, and judgment to scenarios, validating that annotated data mirrors real-world situations accurately. By leveraging the capabilities of both subject matter experts and automated image annotation tools, we can enhance the overall quality and reliability of annotated datasets for AI applications.
Leverage Hybrid Intelligence (AI + Human Expertise) for Superior Outcomes
Let our professionals handle your project requirements with precision and efficiency.